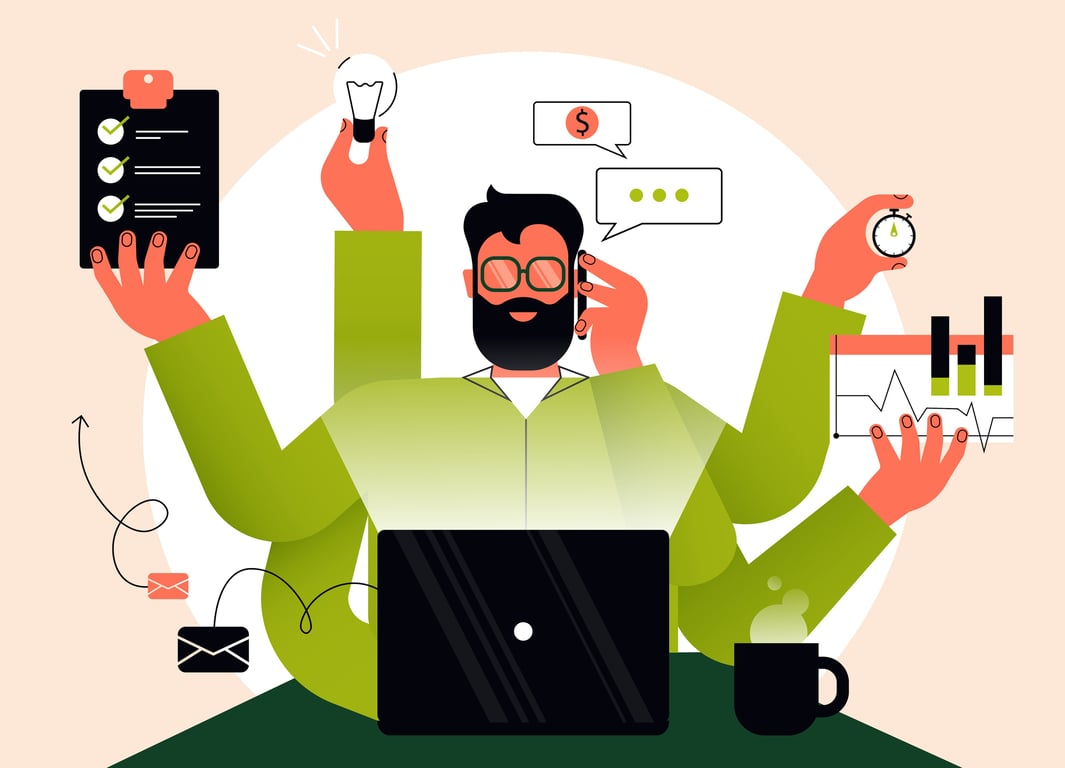
After spending years working with both enterprise companies and startups on their AI initiatives, I've noticed a recurring question that keeps surfacing: "Should we hire a full-time AI engineer or work with consultants?" It's a crucial decision that can significantly impact a company's AI journey, and I'd like to share my perspective based on my experience in the field.
AI Engineer VS AI Consultant
First, let's look at what are AI engineer and AI consultant, and what each of the role is doing.
An AI engineer is essentially a technical specialist who lives and breathes artificial intelligence. They're the ones who roll up their sleeves to deploy open-source language models, fine-tune existing models, and develop machine learning solutions from scratch. They're incredibly valuable for companies whose primary product is AI-based.
On the other hand, AI consultants take a more holistic approach. In my consulting work, I've found that successful AI implementation is more about understanding business needs and finding the right solutions than it is about pushing technical boundaries. Consultants bridge the gap between cutting-edge AI technology and practical business applications, helping companies navigate the complex landscape of AI integration.
Why Choose AI Consulting Over Full-time AI Engineers?
Reason #1: The Experimental Nature of AI Projects
AI projects are inherently experimental, and smart business leaders understand the importance of validating ideas before making substantial investments. In my experience, companies typically want to minimize their initial spending while testing whether their AI concept is viable and valuable. This is where consulting proves invaluable.
Working with consultants allows you to quickly build and test a minimum viable product (MVP) with a relatively small budget. Once you've validated the concept with real users or customers, or secured funding from investors based on demonstrable results, you can make a more informed decision about scaling up the project. I've seen numerous startups successfully use this approach - they start with a consulting team to build a proof of concept, and only after securing funding or validating market demand do they consider building an in-house AI team.
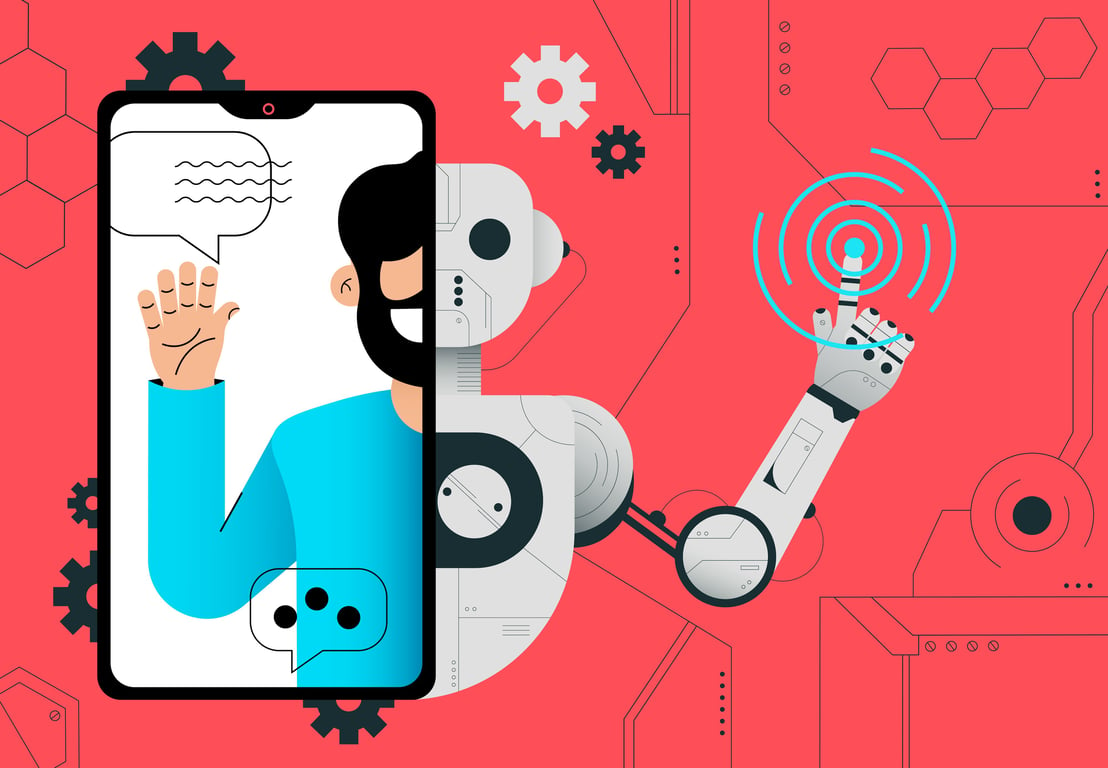
I remember working with a manufacturing company that initially wanted to build their own language model. Through a short consulting engagement, we were able to create a prototype using existing AI services at a fraction of the cost. This allowed them to test their concept with actual users, gather valuable feedback, and ultimately discover that a simpler solution would better serve their needs. Had they immediately hired a full AI team, they would have committed significant resources before validating their assumptions.
Reason #2: Cost-Effectiveness
The cost factor is another crucial consideration. In my experience, most successful AI projects don't actually require building and maintaining custom language models. Here's a reality check that I often share with my clients: deploying an open source LLM on cloud platforms like AWS isn't the cost-saver many expect it to be. The computing resources required for running these models can easily cost $10,000-15,000 per month – essentially equivalent to hiring another full-time engineer. And that's just the infrastructure cost, not including the engineering time needed for deployment, maintenance, and optimization.
Even highly successful AI startups follow this practical approach. Take Leonardo.ai and Perplexity.ai for example - these companies didn't start by building their own models. They strategically launched their products using OpenAI's APIs, focusing on building great products and acquiring users. Only after securing massive funding rounds did they begin investing in developing their own LLM infrastructure. This is a smart strategy I often recommend to my clients: start with proven external APIs, validate your business model, and only consider building your own models when you have significant resources and a clear business case for doing so.
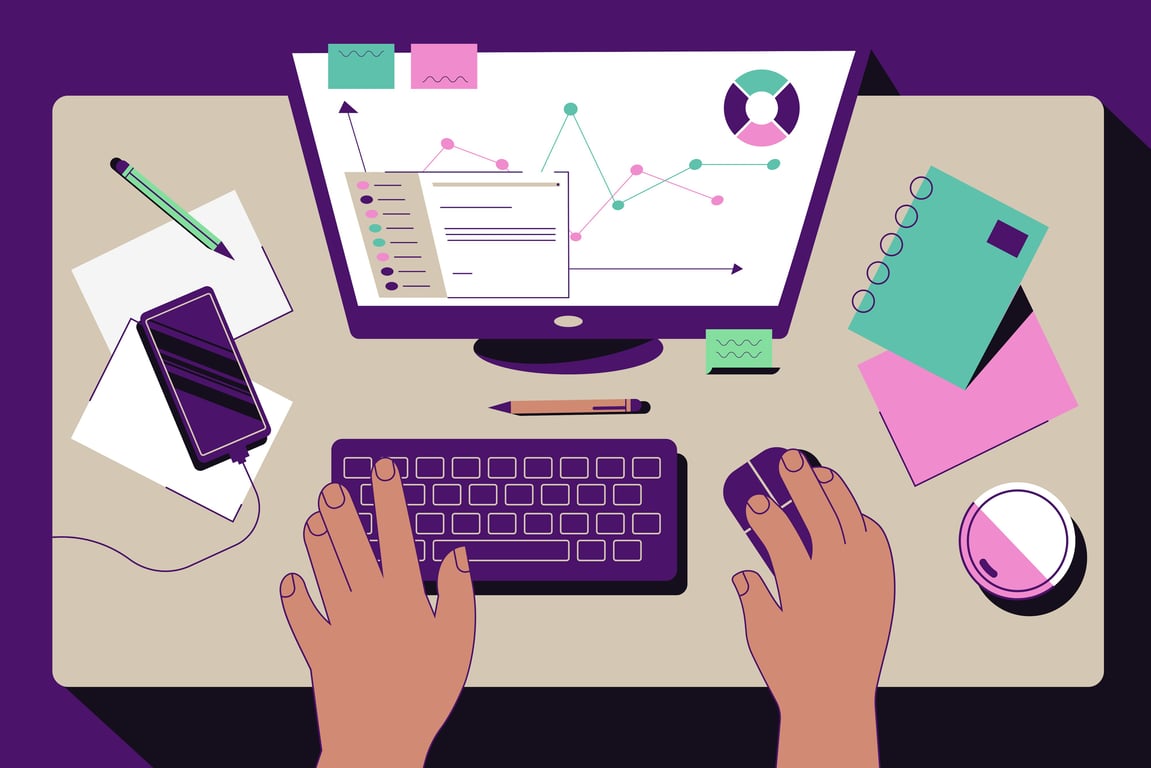
The truth is, companies like OpenAI and Anthropic have invested billions in developing their models, and it's extremely challenging (and expensive) for individual companies to match that quality. Even when successfully deploying open source alternatives, the performance gap compared to leading providers like GPT-4 or Claude is significant. I've seen companies struggle for months trying to fine-tune open source models, only to achieve results that still fall short of what's available off-the-shelf from major providers.
I've found that leveraging these existing services through consulting expertise often provides better results at a fraction of the cost of maintaining an in-house AI team. Why spend resources reinventing the wheel when you can access superior technology at a pay-per-use rate? This approach allows companies to allocate their budget more efficiently, focusing on their core business objectives rather than the complexities of AI infrastructure.
Reason #3: Business-First Approach
This brings me to what I consider the most compelling argument: most AI projects are fundamentally about business transformation, not pushing the boundaries of AI technology. In my work, I've repeatedly seen that companies primarily want to make better use of their proprietary data, whether it's serving their employees or customers more effectively. This is where a technique called RAG (Retrieval-Augmented Generation) comes into play.
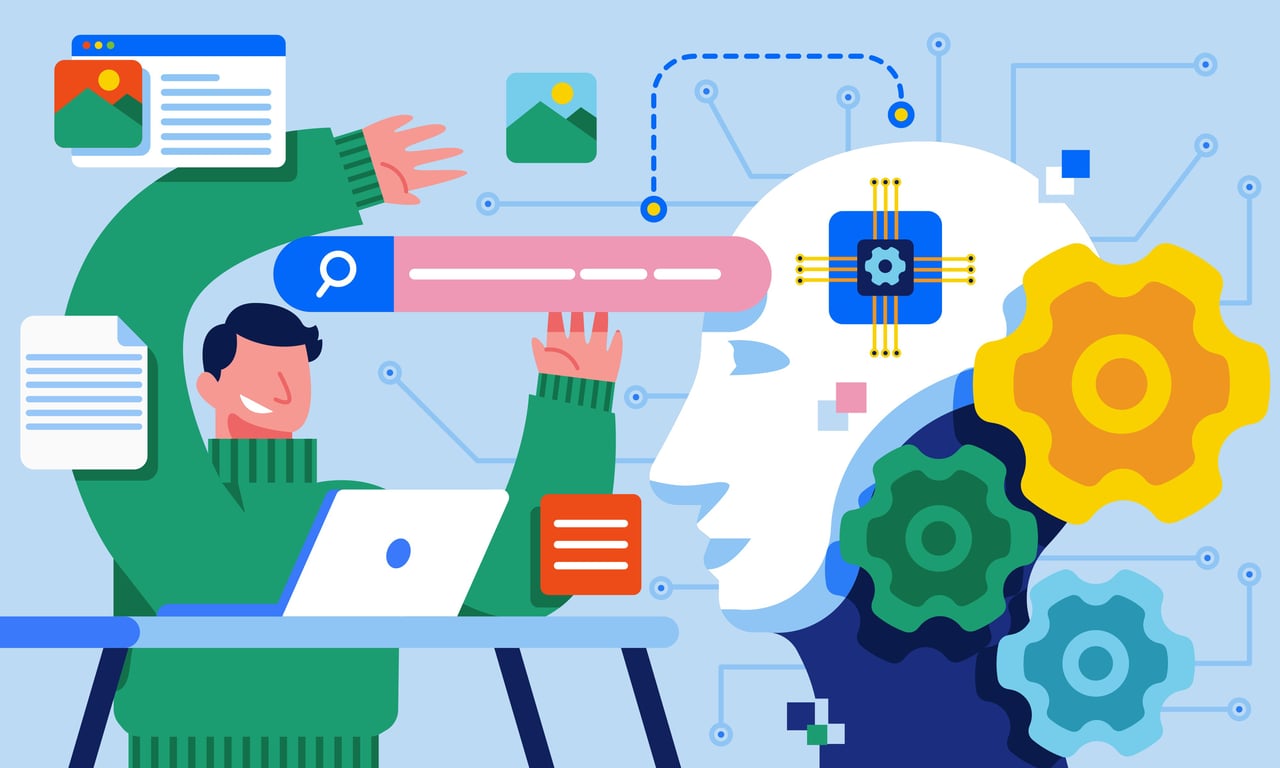
Let me explain RAG (Retrieval-Augmented Generation) in simple terms. Imagine you have a vast library of company documents, policies, and data. RAG is like having a highly intelligent assistant who can instantly access this library, understand the content, and provide accurate, contextual responses using the power of large language models. The beauty of RAG is that it allows companies to leverage advanced AI capabilities while keeping their sensitive data secure and private.
I've implemented RAG solutions for various clients, and the results have consistently shown that this approach is more practical than fine-tuning models. One of my clients, a legal firm, was initially convinced they needed to fine-tune their own language model for legal document analysis. However, after implementing a RAG-based solution using existing AI providers, they achieved better results in less time and at a lower cost.
Addressing the Data Privacy Challenge
Now, I know what you might be thinking: "What about data privacy when using external LLM providers?" This is a valid concern that I encounter frequently in my consulting work. Many companies, especially those dealing with sensitive information like healthcare records or financial data, are rightfully cautious about using external AI services.
As an AI consultant, I've developed and implemented various strategies to address these privacy concerns. One of the most effective approaches I use is data desensitization - a process where we carefully remove or mask sensitive information before sending any data to external LLMs. This might involve anonymizing personal identifiers, removing confidential business information, or restructuring data to maintain privacy while preserving the context needed for analysis.
I'm also excited to see the AI industry actively working on this challenge. For instance, Anthropic's Claude AI has made significant strides in handling data more securely, and many providers are developing sophisticated privacy-preserving techniques. These developments mean that companies can increasingly benefit from state-of-the-art AI capabilities while maintaining robust data protection.
The Path Forward
Of course, there are exceptions to this recommendation. If you're building an AI-first product or your core business revolves around developing novel AI technologies, then yes, you absolutely need full-time AI engineers. But for most businesses, especially those in traditional industries looking to modernize their operations, I've found that working with AI consultants provides the best balance of expertise, flexibility, and cost-effectiveness.
What I always tell my clients is this: start with your business objectives, not the technology. Work with consultants who understand both the technical capabilities and privacy requirements of your industry. A good AI consultant will help you navigate these challenges and design solutions that balance innovation with data protection. This approach has consistently led to more successful, sustainable AI integrations that deliver real business value while maintaining the highest standards of data privacy.